数据创造价值
To create the maximum value, urgency, and leverage in a data partnership, you must present the data available for sale or partnership in a clear and comprehensive way. Partnerships are based upon the concept that you are offering value for value, whether paid or traded. Friendship might need no reasons, but partnerships require some understanding of the exchange of value.
要在数据合作伙伴关系中创造最大价值,紧迫性和杠杆作用,您必须以清晰,全面的方式显示可用于销售或合作伙伴关系的数据。 伙伴关系基于您提供物有所值的概念,无论是付费还是交易。 友谊可能不需要任何理由,但是伙伴关系需要对价值交换有所了解。
The most common way to demonstrate the value of data is to share three different files or documents, each with a slightly different view of your data assets. The first file, called the data brief, is a presentation or document describing your data assets highlighting their best qualities and potential uses, or case studies of actual use. Your best strategy is to present this in person or, at if that’s not possible, in a conversation. The second file is a comprehensive document called a data catalog that outlines all of the facts about your data assets. The last file is a sample data file to assist would-be partners to test your data. The brief, catalog, and sample files need to represent accurately and clearly the value of your data.
证明数据价值的最常见方法是共享三个不同的文件或文档,每个文件或文档的数据资产视图略有不同。 第一个文件称为数据摘要,是一个描述或文档,描述了您的数据资产,突出显示了它们的最佳质量和潜在用途,或实际用途的案例研究。 最好的策略是亲自展示,或者在不可能的情况下通过对话展示。 第二个文件是一个称为数据目录的综合文档,概述了有关数据资产的所有事实。 最后一个文件是样本数据文件 ,可帮助潜在的合作伙伴测试您的数据。 简要,目录和样本文件需要准确,清楚地表示数据的价值。
Companies that fail to present data assets effectively will also fail to attract partnerships and receive fair compensation for their data. The value of your data is not obvious — just ask anyone in your company who doesn’t directly work with a given dataset to evaluate its purpose and worth to see what we mean. And those who do work directly with the data often can’t give a solid business case, either. This means, unfortunately, that those closest to the data, meaning you and your team, are also those most likely to become frustrated with a potential partner’s misunderstanding the value of your data. To prevent that frustration, invest the time to create the brief, the catalog, and the sample file.
未能有效展示数据资产的公司也将无法吸引合作伙伴,也不会为其数据获得公平的补偿。 数据的价值并不明显-只需让公司中不直接使用给定数据集的任何人评估其目的并值得了解我们的意思。 而那些谁直接做的工作与数据往往不能给出一个稳固的业务情况下,无论是。 不幸的是,这意味着那些最接近数据的人,也就是您和您的团队,也最有可能因潜在合作伙伴误解您的数据价值而感到沮丧。 为避免这种麻烦,请花时间创建摘要,目录和示例文件。
证明数据价值的三个文件 (The Three Files That Prove the Value of your Data)
The brief, the initial presentation file to highlight your data assets, need not be very long. To get potential customers or data partners to value the data appropriately, you should present it in simple terms, with appealing visuals that highlight the data assets clearly. Show the data at its most interesting, intriguing, exciting.
简短的初始演示文件可以突出显示您的数据资产,不需要很长。 为了使潜在的客户或数据合作伙伴适当地评估数据,您应该以简单的术语来呈现它,并用吸引人的视觉效果清晰地突出显示数据资产。 以最有趣,最有趣,最令人兴奋的方式显示数据。
The brief needs to start with a simple definition of your dataset that describes it clearly, for example:
简要说明需要从对数据集的简单定义开始,以清楚地描述它,例如:
- The full semantic breakdown of the United States Patent library. 美国专利图书馆的完整语义分类。
- The only user-generated, real-time gas price tracking platform. 唯一的用户生成的实时天然气价格跟踪平台。
- The deepest collection of opt-in consumer profiles for luxury sales. 参与奢侈品销售的最深入的选择加入的消费者档案。
- The highest resolution, hourly drone footage of US retail store locations. 美国零售商店位置的最高分辨率,每小时无人机画面。
Each of these sentences describe real datasets in their most appealing value terms. Focusing on the most important attributes like “user-generated,” “real-time,” or “highest resolution” are meant to highlight how they are both unique and valuable.
这些句子中的每一个都以其最吸引人的价值术语描述了真实的数据集。 关注“用户生成”,“实时”或“最高分辨率”等最重要的属性是为了突出它们的独特性和价值。
From here, your presentation should draw out each major section of your data catalog, which is the second file you must create. Use striking graphics or “hero” numbers that clearly demonstrate the highlights in your data, such as the total number of records, how often the data is updated, quality metrics, and geographic or sector breakdowns. Each dataset has its own unique highlights.
从这里,您的演示文稿应该绘制出数据目录的每个主要部分,这是您必须创建的第二个文件。 使用醒目的图形或“英雄”数字清楚地显示数据中的亮点,例如记录总数,数据更新频率,质量指标以及地理或部门细分。 每个数据集都有其独特的亮点。
In the second file, the data catalog, key sections include the Initial Data Definition, the Method of Data Collection, the Refinement Process, the Commitment of Quality, and the Coverage, Fill Rates, and Refresh Rates per major data field. Your partner will appreciate it if you include a Field Definitions Library at the end of the document, showing each field available, its data format (number, Boolean, date, currency, or text string, for example), its fill quantity (the percentage of records in which the field is filled), and a brief definition of what it conveys.
在第二个文件中,数据目录的关键部分包括“初始数据定义”,“数据收集方法”,“细化过程”,“质量承诺”以及每个主要数据字段的覆盖率,填充率和刷新率。 如果您在文档末尾包含一个字段定义库,它会显示每个可用字段,其数据格式(例如数字,布尔值,日期,货币或文本字符串),填充量(百分比),那么您的合作伙伴将不胜感激。填充该字段的记录),以及对其传达的内容的简要定义。
Your business and data teams should outline the initial brief as well as the data catalog, and your marketing department or agency should design them. Of course, for many companies, that means a single person doing the work, and so be prepared to allocate the resources necessary to give that person the support needed. This investment in clearly articulating what data assets you have will drastically shorten the partnership or sales cycle, immediately showcasing your data assets at a higher level than most.
您的业务和数据团队应概述初始摘要以及数据目录,营销部门或代理商应设计它们。 当然,对于许多公司而言,这意味着只有一个人在从事这项工作,因此要做好分配必要资源的准备,以便为该人提供所需的支持。 明确说明您拥有哪些数据资产的这项投资将大大缩短合作伙伴关系或销售周期,并立即以高于大多数的水平展示您的数据资产。
Many companies shortchange this effort and as result, never receive full value for their data. When you jump right to “let us send you a sample file” you miss the opportunity to build up your data value and control the dialogue. You also likely keep the decisionmaking at a lower level of authority with your prospective partner — a poorly explained proposal will get handed off to someone who isn’t a key decisionmaker, adding a level of delay and uncertainty to the process.
许多公司都缩短了工作量,结果是永远无法获得其数据的全部价值。 当您跳到“让我们给您发送示例文件”时,您会错过建立数据价值和控制对话的机会。 您也可能会与潜在的合作伙伴保持较低的决策权限-一个解释不充分的建议会交给非关键决策者,这会增加流程的延迟和不确定性。
You should create the final file, the sample, dynamically based upon the circumstance. While it’s not usually necessary to customize the brief or the catalog, a custom sample data file will give each potential partnership its best chance at success. For example, for the drone footage company mentioned above, if they are meeting with a major electronics retailer, may want to specifically and only show similar footage or population density visuals for similarly situated electronics retailers. If the sample dataset has restaurants and laundromats in it, this will reveal shortcomings to the potential buyer or partner. They may show that the depth or coverage of the data file is inadequate, or maybe signal that the data owners are unable to deliver a custom data set quickly and in a format that is usable.
您应该根据情况动态创建最终文件(样本)。 尽管通常不需要自定义简介或目录,但自定义示例数据文件将为每个潜在的合作伙伴提供最大的成功机会。 例如,对于上述无人机素材公司,如果他们要与一家主要的电子产品零售商会面,则可能希望专门为那些位置相似的电子产品零售商展示类似的素材或人口密度视觉效果。 如果样本数据集中有餐厅和自助洗衣店,这将向潜在的买家或合作伙伴揭示缺点。 它们可能表明数据文件的深度或覆盖范围不足,或者可能表明数据所有者无法以可用格式快速交付自定义数据集。
Most companies that are considering a data partnership or the purchase of data assets will not have any patience for file delivery or formatting issues. This means that you should be prepared with a file prior to your first meeting that is likely to be appropriate to your audience, but also have a plan to quickly generate a different file if the meeting indicates a different need.
大多数正在考虑建立数据合作伙伴关系或购买数据资产的公司将对文件传递或格式问题没有任何耐心。 这意味着您应该在第一次会议之前准备好可能适合您的听众的文件,但也要制定计划,以便在会议表明有不同需求时快速生成另一个文件。
关系映射值 (Relationship Mapping Values)
The process of mapping relationships between your data values and those of the potential partner can cause countless misunderstandings and unnecessary delays. This is typically because each party has very unique ways of looking at a business or person or product in their data; they need a Rosetta Stone of sorts to understand your dataset in their own context. This is central to the entire data partnership strategy for a business because there always needs to be a way to “crosswalk” from one dataset to another. Here, we’ll show how to highlight your data catalog to streamline this process in each partnership discussion.
在数据值与潜在合作伙伴的数据值之间映射关系的过程可能会引起无数的误解和不必要的延迟。 通常,这是因为各方都有非常独特的方式来查看其数据中的企业或个人或产品; 他们需要某种Rosetta Stone才能在自己的环境中理解您的数据集。 这对于企业的整个数据合作伙伴关系战略至关重要,因为始终需要一种从一个数据集“穿越”到另一个数据集的方法。 在这里,我们将在每次合作伙伴讨论中展示如何突出显示数据目录以简化此过程。
Data, in any form, is the reduction of the inputs from the world to values or ranges, so we can efficiently understand and analyze information. In other words, data is a description of, or the story of, our world. As such, every dataset can be related to any other dataset by identifying its key value when it comes to the classic questions of Who, What, When, Where, or Why. Just as in your first journalism class or writing class, these five factors are common ways to describe the world around us and to connect all of the elements of a story together.
任何形式的数据都是从世界输入减少到值或范围,因此我们可以有效地理解和分析信息。 换句话说,数据是对我们世界的描述或故事。 这样,在涉及谁,什么,何时,何地或为什么的经典问题时,通过标识其关键值,可以将每个数据集与任何其他数据集相关联。 就像在您的第一个新闻班或写作班上一样,这五个因素是描述我们周围世界并将故事的所有要素联系在一起的常用方法。
By asking the following questions, both of your own data assets, and then of your potential data partner, you can quickly come to a common language to compare and analyze your data.
通过问您自己的数据资产以及潜在的数据合作伙伴以下问题,您可以快速使用通用语言来比较和分析数据。
- Who is your data about? 您的资料涉及谁?
- What is your data about? 您的数据是关于什么的?
- When did your data occur or change? 您的数据何时出现或更改?
- Where (what location) is your data about? 您的数据在哪里(什么位置)?
- Why was your data created? 为什么创建数据?
Is your data about people, products, or places? If so, each of those can be related to other databases with those as central themes. Consumer profiles, business locations, product codes, and medical reimbursement codes are all examples of common data anchors by which different datasets can be matched and compared. This means that Who, What, and Where are the most straightforward questions to answer, and you or your potential data partner can usually match corresponding data elements so that they can compare your data to theirs and analyze its value. Every data record needs to correspond to the same person, product, or place across datasets.
您的数据是关于人,产品或地点的吗? 如果是这样,那么每个主题都可以与其他数据库相关并以它们为中心主题。 消费者资料,营业地点,产品代码和医疗报销代码都是通用数据锚点的示例,通过它们可以匹配和比较不同的数据集。 这意味着,谁,什么和哪里是最容易回答的问题,您或您的潜在数据伙伴通常可以匹配相应的数据元素,以便他们可以将您的数据与其数据进行比较并分析其价值。 每个数据记录都需要在数据集中对应同一个人,产品或地点。
Time is another fantastic way to connect data assets. When two data sets don’t describe the same person, product, or place, time of occurrence or change is the next most likely area of correspondence. This is how drone footage and satellite imagery are tied to product sales at a retail store, for example. Both the photos of the cars or pedestrians in the parking lot of a retail store can be compared to transactional data from the store, because both have time stamps. While photos of parking lot density can’t directly be tied to a product SKU, they can be compared to the times a particular product or series of products is purchased. Time is a universal connector that powerfully connects seemingly disparate datasets.
时间是连接数据资产的另一种绝佳方式。 当两个数据集无法描述同一个人,产品或地点时,发生或更改的时间是下一个最可能的对应区域。 例如,这就是将无人机画面和卫星图像与零售商店的产品销售联系起来的方式。 零售商店的停车场中的汽车或行人的照片都可以与商店的交易数据进行比较,因为两者都有时间戳。 虽然不能将停车场密度的照片直接与产品SKU相关联,但可以将其与购买特定产品或一系列产品的时间进行比较。 时间是一个通用连接器,可以有效地连接看似完全不同的数据集。
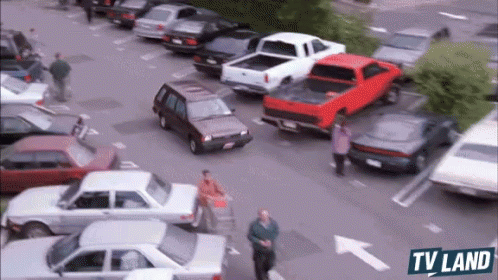
The last and most complicated data relationship to map, in both data and in writing, is around the question of “why.” The best way to think about how this matches up with other datasets is to try to match up sentiment or indications of interest around an event, product, or service. For example, many firms like Yelp semantically extract the sentiment of reviews left by patrons at restaurants and hotels, sentiments that can help answer the question of “why.” When a reviewer of a restaurant leaves a comment like, “You have to try the 1-pound meatball appetizer, it’s amazing,” they can relate the object of the meatball to a positive consumer experience and the subsequent recommendation of that business to others. This is one of the hardest elements of data collection, but the increasingly easy access to user interest and sentiment through mobile phone apps has created a whole new world of “why” relationship mapping.
在数据和书面形式上,映射的最后也是最复杂的数据关系是围绕“为什么”的问题。 考虑这与其他数据集如何匹配的最好方法是尝试围绕事件,产品或服务来匹配情绪或兴趣指示。 例如,许多类似Yelp的公司从语义上提取了顾客在餐馆和酒店留下的评论情绪,这些情绪可以帮助回答“为什么”的问题。 当餐厅的评论者留下诸如“您必须尝试1磅重的丸子开胃菜,真是太神奇了”的评论时,他们可以将丸子的目标与积极的消费者体验联系起来,并将该业务随后推荐给其他人。 这是数据收集中最困难的要素之一,但是通过手机应用程序越来越容易获得用户的兴趣和情感,这为“为什么”关系映射开辟了一个全新的世界。
时间数据 (Data Through Time)
When presenting data assets, reviewing changes over time is a powerful magnifier of value. Charts of daily, weekly, monthly, quarterly, or annual shifts put your data assets into a common perspective. Most data briefs and data catalogs show the growth of their data assets — records, fields, or fill rates — as time-based series. Since most data collection efforts are cumulative, they grow over time, which attractively demonstrates their value. For this reason, you should identify each dataset you have, how many, and when they were created to make this very clear. Charting growth over time can also be a great way to highlight that your lead over competing data suppliers, and how long it would take to recreate your data at the same scale. For this reason, your brief and data catalog should convey how your scale or head-start create dominance in your space.
在显示数据资产时,回顾随时间的变化是一个强大的价值放大器。 每日,每周,每月,每季度或每年的变化图表将您的数据资产置于一个共同的角度。 大多数数据摘要和数据目录以时间为基础的系列显示其数据资产(记录,字段或填充率)的增长。 由于大多数数据收集工作都是累积性的,因此它们会随着时间的推移而增长,这很有吸引力地证明了它们的价值。 因此,您应该确定每个数据集,数量以及创建时间,以使其更加清晰。 绘制一段时间内的增长图表也可以很好地说明您在竞争数据供应商方面的领先优势,以及以相同规模重建数据需要花费多长时间。 因此,您的简要和数据目录应该传达您的规模或先发优势如何在您的空间中创造支配地位。
Another important temporal view of your data will reveal changes or updates to your data and when they occur. To highlight just how dynamic your data assets are, create scatter plot diagrams that show fields, how often they update, and to what magnitude they change. This insight can visually help a potential partner to understand not just the need for your data, but the need for refreshing their feed of data from you on a timely basis. Some data suppliers will want to convey this early on in a discussion, because their potential data partners will have trouble ingesting data frequently. Partners’ legacy corporate data structures and slow compilation practices can create barriers where, even if your data is amazingly useful, a data partner may not be able to ingest it fast enough to use it properly. This is why a chart of the frequency of updates is very helpful early, enabling you to demonstrate your capabilities to a potential data partner. Frequency charts also help your partner understand just how large of a data inflow they may be purchasing — It is a sad discussion when a data partnership is abandoned after weeks of discovery only because the receiving party realizes they can’t ingest the fire hose of content you might be able to provide.
数据的另一个重要时态视图将揭示数据的更改或更新以及发生的时间。 要突出显示数据资产的动态性,请创建散点图,以显示字段,字段更新的频率以及更改的幅度。 这种见解可以从视觉上帮助潜在的合作伙伴不仅了解您的数据需求,而且还需要及时刷新您的数据源。 一些数据提供者将希望在讨论中尽早传达这一点,因为它们的潜在数据合作伙伴将难以频繁提取数据。 合作伙伴的旧公司数据结构和缓慢的编译实践可能会造成障碍,即使您的数据非常有用,数据合作伙伴也可能无法足够快地提取数据以正确使用它。 这就是为什么更新频率图表在早期非常有用的原因,它使您能够向潜在的数据合作伙伴展示自己的能力。 频率图表还可以帮助您的合作伙伴了解他们可能购买了多少数据流入—当发现数周后放弃数据合作伙伴关系而仅仅是因为接收方意识到他们无法摄取内容的消防水带时,这是一个悲伤的讨论您可能能够提供。
We mentioned the need for storing the date and time for each update in your data. This is where that effort really shines in the data partnership strategy. Work with your technology teams to ensure that creation, updates, changes, confirmations, and deletions of every data field are tracked and time-stamped. This will be a hallmark of your data value presentation.
我们提到需要在数据中存储每次更新的日期和时间。 这就是这种努力真正体现在数据合作伙伴战略中的地方。 与您的技术团队合作,以确保跟踪和标记每个数据字段的创建,更新,更改,确认和删除。 这将是您的数据值表示的标志。
Ultimately, the vale of a dataset is only apparent when explained, because anyone who has not directly worked with the data will only recognize the value that is surface-level. It takes time, energy, and creativity to produce an explanation of data that will convince a prospective partner of value. But the investment in making the data brief, data catalog, and sample data file will be well worth the resources required. A weak presentation has been the downfall of many a potential partnership; a strong presentation goes a long way to showing why your data is worth your partner’s time, and yours.
最终,数据集的价值只有在解释时才显而易见,因为任何未直接处理数据的人都只会识别表面值。 对数据进行解释需要花费时间,精力和创造力,这些数据才能使潜在的合作伙伴信服价值。 但是,使数据简短,数据目录和样本数据文件方面的投资非常值得所需的资源。 表现不佳是许多潜在伙伴关系的失败。 出色的演示对显示为什么您的数据值得您的伴侣和您的伴侣的时间大有帮助。
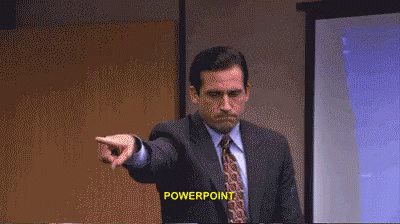
Originally published at https://wardpllc.com on September 4, 2020.
最初于 2020年9月4日 在 https://wardpllc.com 上 发布 。
Gain Access to Expert View — Subscribe to DDI Intel
获得访问专家视图的权限- 订阅DDI Intel
翻译自: https://medium.com/datadriveninvestor/presenting-data-and-creating-value-dec9fffb514c
数据创造价值
本文来自互联网用户投稿,该文观点仅代表作者本人,不代表本站立场。本站仅提供信息存储空间服务,不拥有所有权,不承担相关法律责任。如若转载,请注明出处:http://www.mzph.cn/news/389297.shtml
如若内容造成侵权/违法违规/事实不符,请联系多彩编程网进行投诉反馈email:809451989@qq.com,一经查实,立即删除!