科学价值 社交关系 大数据
A crucial part of building a product is understanding exactly how it provides your customers with value. Understanding this is understanding how you fit into the lives of your customers, and should be central to how you build on what already exists. It is a way of ensuring that every decision taken will be positive and ultimately improve the value you deliver.
构建产品的关键部分是准确了解产品如何为客户提供价值。 了解这一点就是了解您如何适应客户的生活,并且应该成为您如何基于已有资源构建的核心。 这是确保所做出的每个决定都是积极的并最终提高您提供的价值的一种方法。
In the last couple of months the value question has been hot on the lips of some of us at Jeff. Considering the current global situation and the resulting difficulty of continuing to expand a rapidly growing platform, it was a good moment to take a step back and really think about how our customers were taking advantage of “the good, good life”.
在过去的几个月中,价值问题一直困扰着我们杰夫(Jeff)的某些人。 考虑到当前的全球形势以及持续扩展快速增长的平台所带来的困难,现在是退后一步,真正考虑我们的客户如何利用“美好,美好生活”的好时机。
我们如何到达这里 (How we got here)
The company wide project started with the new user experience team, who got data science involved after some early conversations. Understanding our service’s value was one of their first initiatives, and they saw that a necessary part of this was drawing the typical “customer journey”. These are the typical life cycles that users have on the platform and they help us clearly pinpoint the different moments where customers are delivered value. To draw this they needed a general overview of our different users’ behaviours.
公司范围内的项目始于新的用户体验团队,该团队在进行了一些初期交谈之后就参与了数据科学的研究。 了解我们服务的价值是他们的首批举措之一,他们认为其中的必要部分正在吸引典型的“客户旅程”。 这些是用户在平台上拥有的典型生命周期,它们可以帮助我们明确指出为客户交付价值的不同时刻。 为此,他们需要对我们不同用户的行为进行总体概述。
Getting in touch with the data science team to see if we could demystify some aspects, everyone quickly realised that one of the biggest challenges facing us was that most of the understanding of our company was dispersed, based on intuition, and not easily accessible. Hypotheses were unconfirmed, and complex topics were relatively unexplored. This is pretty typical for many organisations, especially when they are still young, rapidly changing, or don’t have a strong research culture.
与数据科学团队联系,看看我们是否可以揭开某些神秘面纱,每个人都Swift意识到,我们面临的最大挑战之一是,基于直觉,对我们公司的大多数理解是分散的,并且不易获得。 假设尚未得到证实,而相对复杂的话题尚未得到探讨。 这对于许多组织来说是非常典型的,尤其是当它们还很年轻,变化Swift或者没有强大的研究文化时。
While this made the task at hand more difficult, it also has several repercussions for decision making. The first main issue is that teams are mostly blind to research not done by themselves, and are doomed to either waste time on ad hoc investigations, miss out on what other teams already know, or make decisions for the wrong reasons. The other is a struggle to gauge the impact of any changes to the platform. Should we encourage more users to subscribe? Is that more important than improving onboarding of new users? This is hard to understand on the fly and makes prioritising a vague process.
尽管这使手头的任务变得更加困难,但对决策也有一些影响。 第一个主要问题是,团队大多对自己无法完成的研究视而不见,并且注定要浪费时间进行临时调查,错过其他团队已经知道的知识或出于错误的原因做出决定。 另一个是努力评估平台任何更改的影响。 我们应该鼓励更多的用户订阅吗? 这比改善新用户的加入更为重要吗? 这是很难即时理解的,并且会使模糊的过程成为优先事项。
With both of our teams being relatively young, we saw this as a good opportunity to not only analyse our value proposition, but also to deliver a broad, unified understanding that could be used by anyone in the company when it came to decision making.
由于我们的两个团队都相对年轻,我们认为这是一个很好的机会,不仅可以分析我们的价值主张,还可以提供广泛,统一的理解,供公司中的任何人在决策时使用。
细分客户 (Segmenting our customers)
One of the beautiful things about the data — user experience partnership is that both sides can readily contribute to a common goal in ways that the other cannot.
关于数据的美丽之处之一-用户体验合作关系是,双方可以轻易以双方无法做到的方式为共同的目标做出贡献。
Part of the initial problem was understanding exactly what the status quo was — understanding what users come to us for. This is a daunting task, considering the thousands of different users with all of their peculiarities. As a data scientist however, detecting and quantifying diverse behaviours should be your bread and butter.
最初问题的一部分是确切地了解现状-了解用户向我们寻求什么。 考虑到成千上万的不同用户的特殊性,这是一项艰巨的任务。 但是,作为数据科学家,检测和量化各种行为应该是您的头等大事。
This seemed like a typical case that calls for a user segmentation, which is basically the division of users into different common behaviours. This classic concept is quite simple, but in practice, a good segmentation is nuanced and defined by a central trade off. For it to be useful, we need to design segments that contain specific, uniform behaviours, that all carry some business meaning. The trade off is that many tiny groups, created using all of the variables available to you, are all specific and uniform — but a few big groups designed using only a few company level KPIs are far, far easier to understand and use practically. The bonus difficulty is that there isn’t a single metric that will evaluate the quality of your segments.
这似乎是一个典型的案例,需要进行用户细分 ,这基本上是将用户划分为不同的常见行为。 这个经典概念非常简单,但是在实践中,通过权衡取舍可以很好地细分和定义良好的细分。 为了使其有用,我们需要设计包含特定且统一的行为的细分市场,这些行为均具有一定的业务意义。 需要权衡的是,使用您可用的所有变量创建的许多小型小组都是特定且统一的,但是仅使用几个公司级KPI设计的几个大型小组实际上就容易得多,而且更容易理解和使用。 额外的困难在于,没有一个可以评估细分受众群质量的指标。
This problem typically arises when data scientists are too quick to shove a whole database into their favourite algorithm. In our particular case, the platform combines online, offline, subscribers, and occasional users — without even mentioning our other customers, the franchise owning partners — a lot of combinations and the need to create a more or less unified framework. Considering that to start with we were interested in a general overview of behaviour, we alleviated the dilemma by focusing on variables that reflect the core of the business, and by creating the segments focusing on interpretable “cut off” points — the limits we used to define different behaviour groups. All of this while taking into account the entire lifecycle of our users on the platform.
当数据科学家太快而无法将整个数据库推入他们喜欢的算法时,通常会出现此问题。 在我们的特殊情况下,该平台将在线,离线,订户和偶尔的用户结合在一起-甚至没有提及我们的其他客户,特许经营拥有者-很多组合,而且需要创建或多或少统一的框架。 考虑到我们首先对行为的总体概况感兴趣,因此我们通过关注反映业务核心的变量并创建关注可解释的“截止”点的细分市场(我们过去的限制)来缓解困境。定义不同的行为组。 所有这些都考虑了平台上用户的整个生命周期。
Two good examples are the frequency and number of orders for a user. The frequency is easily divisible into interpretable “categories”, like users who order once a week or once a month — especially since this lines up with how our subscriptions work. Looking at how many users followed different behaviours, we can easily make more or fewer frequency segments like this. The number of orders was a bit more complicated. We saw that the more orders a user had, the more likely they were to be retained long term, but only marginally. Comparing users with more than 5 and 20 orders, for example, we saw that while users with more than 5 were slightly less likely to churn, there were way more of them than those with over 20. We accepted this trade off to define a “retained users” segment that had plenty of customers, only marginally losing out on uniformity of behaviour.
两个好的例子是用户的订单频率和数量。 频率很容易划分为可解释的“类别”,例如每周或每月订购一次的用户-尤其是因为这与我们的订阅工作方式保持一致。 观察有多少用户遵循不同的行为,我们可以轻松地像这样创建更多或更少的频率段。 订单数量稍微复杂一些。 我们看到用户拥有的订单越多,则越有可能长期保留订单,但仅保留一部分订单。 例如,比较订单数量超过5和20的用户,虽然用户数量超过5的用户流失的可能性略小,但与用户数量超过20的用户相比,用户流失的可能性更大。我们接受了这种权衡来定义“拥有大量客户的“保留用户”细分受众群,但在行为统一性方面仅微不足道。
This approach meant different behaviour groups are easy to understand and immediately relevant to current strategy, while being defined in a purposeful and meaningful way thanks to our interpretable “cut offs”. For example, it becomes very clear how much we stand to gain from converting new users to “retained” users, the potential target audience for subscription up-selling (frequently ordering users without a subscription), as well as how users’ behaviour evolves (how they move from one segment to another over time).
这种方法意味着不同的行为群体易于理解,并与当前策略直接相关,同时由于我们可解释的“临界值”,以有目的和有意义的方式对其进行了定义。 例如,非常清楚的是,从将新用户转换为“保留”用户,潜在地进行订阅向上销售(经常订购没有订阅的用户)的目标受众以及用户行为的演变,我们将获得多少收益(随着时间的推移,它们如何从一个细分转移到另一个细分)。
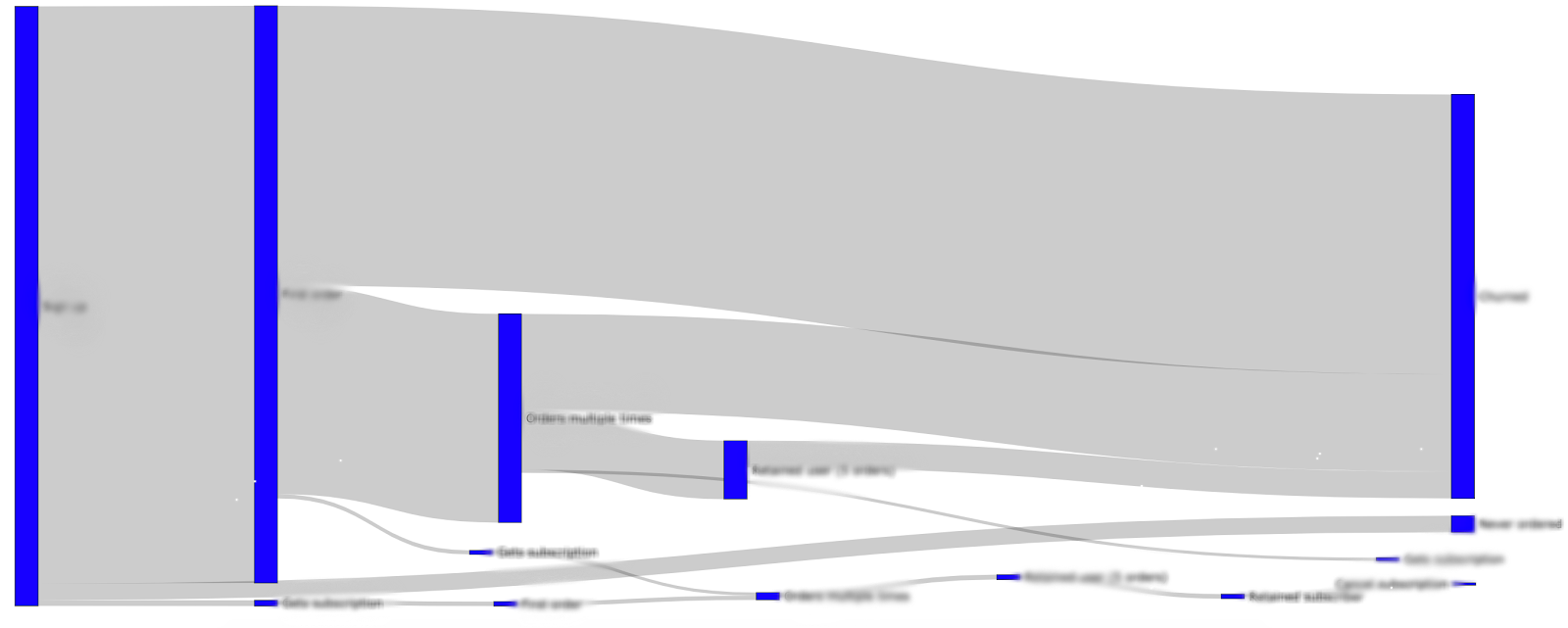
Throughout the process of building our segments, we also adopted a methodology of continuously delivering concrete insights about what we were finding, ranging from how long users take to make their first order, to how much subscribers contribute to the financial health of a hub. These were all key pieces of knowledge that we found to be missing when we started the project. The continuous delivery of these conclusions gave our young team plenty of low hanging fruit to make some quick impact.
在构建细分市场的整个过程中,我们还采用了一种方法,不断对发现的结果提供具体的见解,范围从用户下达第一笔订单所需的时间到订户对枢纽财务状况的贡献有多大。 这些都是我们在开始项目时发现缺少的关键知识。 这些结论的不断传递使我们的年轻团队垂涎三尺,可以Swift产生影响。
The next step specific to our segments is to understand how they can best be used directly to improve the development of new features and strategy. We are also in the process of understanding how they might be useful for our franchise partners, so that they can improve how they run their businesses.
我们细分市场的下一步是了解如何最好地将其直接用于改进新功能和策略的开发。 我们也在了解它们对我们的特许合作伙伴可能有用的方式,以便他们可以改善其业务运作方式。
用户体验和我们的价值主张 (User experience and our value proposition)
With these findings, we were able to get rid of the vague, general context that we started with, and replace it with a detailed, specific overview of the different customer behaviours. This allowed the user experience team to start to really flesh out their understanding of the user journey, adding new paths, expanding on existing ones, and supplementing the design with concrete statistics on behaviour. We could now understand where we are fulfilling our promised value, where we are not, and where users are finding value in unexpected ways.
有了这些发现,我们就摆脱了最初的含糊,笼统的背景,而用不同的客户行为的详细,详细的概述代替了它。 这样,用户体验团队就可以开始真正充实他们对用户旅程的理解,添加新路径,扩展现有路径,并通过行为的具体统计数据来补充设计。 现在,我们可以了解我们在哪里实现了承诺的价值,在哪里没有实现,以及用户在哪里以意想不到的方式找到价值。
Having reached this point, the user experience team is also in a much better position to plan future research activity. They can interview our customers and ask exactly why they do certain things, and deliver the company insights that data science alone could not. It allows us to understand the motivations behind user behaviour, including external factors that affect this.
到此为止,用户体验团队也可以更好地计划未来的研究活动。 他们可以采访我们的客户,并确切询问他们为什么要做某些事情,并提供仅凭数据科学无法做到的公司见解。 它使我们能够了解用户行为的动机,包括影响用户行为的外部因素。
We are confident that this will be the first major step on our way to fully understand how we improve the lives of our customers. By taking advantage of data science’s ability to summarise how users behave, user experience is able to both fully understand the customer journey with the service, as well as round off our overall understanding with their own differential research. This puts us well on the path to understanding what role Jeff plays in the lives of its customers, and will hopefully drive real, research backed improvements to the service in the future.
我们相信,这将是我们全面了解如何改善客户生活的第一步。 通过利用数据科学总结用户行为的能力,用户体验既可以全面了解客户使用该服务的旅程,又可以通过他们自己的差异研究来完善我们的整体理解。 这使我们能够更好地理解Jeff在客户生活中所扮演的角色,并有望在未来推动对服务进行真正的,研究支持的改进。
We will be sure to post an update on how things are going in the near future, but in the meantime don’t hesitate to get in touch if you have any questions, comments, or feedback!
我们一定会发布一个更新的事情是如何在不久的将来打算,但在此期间不要犹豫 取得联系 ,如果您有任何疑问,意见或反馈!
翻译自: https://medium.com/jeff-tech/the-value-of-a-service-data-science-and-user-experience-investigate-the-good-good-life-cdc7044e06a7
科学价值 社交关系 大数据
本文来自互联网用户投稿,该文观点仅代表作者本人,不代表本站立场。本站仅提供信息存储空间服务,不拥有所有权,不承担相关法律责任。如若转载,请注明出处:http://www.mzph.cn/news/388103.shtml
如若内容造成侵权/违法违规/事实不符,请联系多彩编程网进行投诉反馈email:809451989@qq.com,一经查实,立即删除!