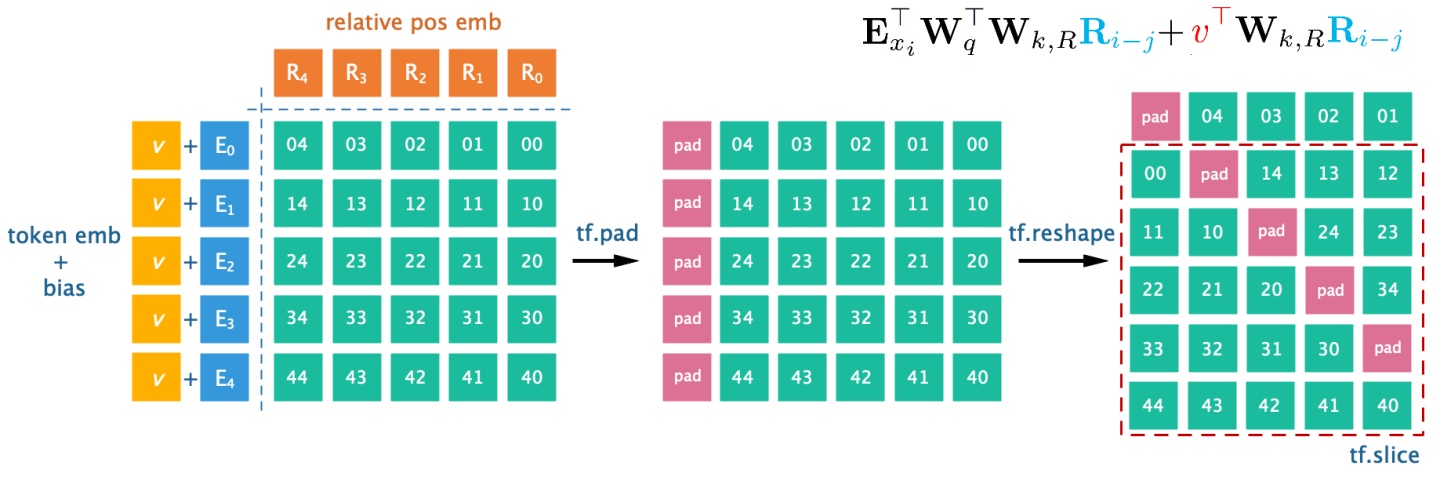
[论文]Transformer-XL: Attentive Language Models Beyond a Fixed-Length Contextarxiv.org
Motivation
- Transformer在预训练阶段,设置了固定序列长度
max_len
的上下文,finetuning阶段,模型不能获取大于max_len
的上下文依赖; - Transformer在长文本编码过程中,可采用按照句子边界拆分和按照
max_len
截断的方式,进行片段的编码,论文指出第二种不考虑句子边界的编码方式效果更好,但仍然存在上下文碎片的问题context fragmentation
。
How to solve
- Segment-level recurrence mechanism - 使模型获取更长上下文
在单向编码长文本时,由于transformer设置了max_len
,从而在训练时,第i
个token只能attention到前i
个token,且当transformer以max_len
为窗口滑动时,每前进一步,绝对位置编码需要跟着前进一步,使得每一步都需要重新计算max_len
中每个token的隐层表示,如下图。
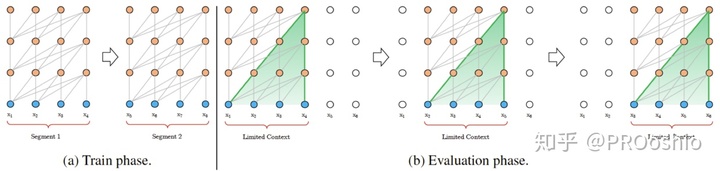
Transformer-XL通过设置memory-span
使得当前max_len
窗口中的每个token都能attention到前max_len
个token,因此Transformer-XL在每前进一步时,只用计算当前位置的token的隐层表示,同时在更新梯度时,只更新当前窗口内的梯度(防止梯度bp的距离太远),从而实现了输出隐层表示的更长上下文关联,和高效的编码速度。
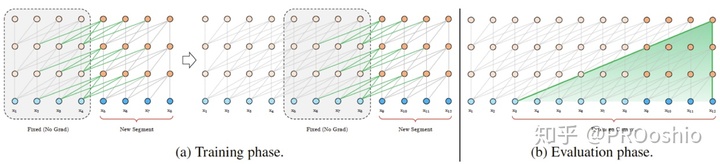
- Relative Positional Encodings - 使模型在前向过程中更快
Segment-level recurrence mechanism
机制中提到的,max_len
窗口中的每个token都能attention前max_len
个token,其中可能会有一些token在上一个seg,这样就存在位置编码不连续,或者相同token在当前seg和前一个seg具有相同的attention值的问题。因此为了实现transformer-XL训练和长文本编码运用之间的等效表示,将绝对位置编码替换为以当前token为基准的相对位置编码Relative positional encodings
。
- 绝对位置编码 - attention-score

- 相对位置编码 - attention-score

其中
token emb, absolute pos emb, relative pos emb, proj matrix
,对于每个编码的token相对位置编码都为0
,因此 绝对位置编码在输入transformer之前就和token emb
求和,相对位置编码需要在计算attention score
加入和计算。在Transformer-XL的tensorflow代码是如何实现呢?
Relative positional emb 代码解析
- 在解析代码前,先用图示展示relative pos emb的实现过程(无memory版本)
rel_shift(*)
- 输入
token emb
和反向的absolute pos emb
- 得到
attention score
矩阵后,在token emb
维pad,产生1位错位; - 截取位置编码对齐后的矩阵。
- 按顺序截取
token emb
个数个分数组成行,对角全是pad
- 输入
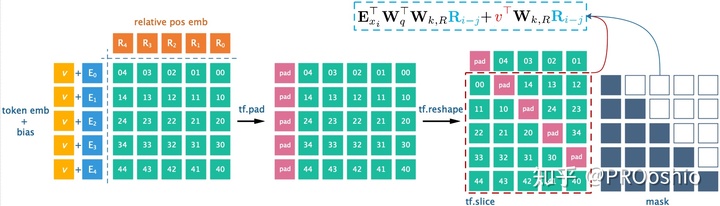
- 在tf中,
rel_multihead_attn
函数生成相对位置的attention score
def rel_multihead_attn(w, r, r_w_bias, r_r_bias, attn_mask, mems, d_model,n_head, d_head, dropout, dropatt, is_training,kernel_initializer, scope='rel_attn')
# w : token emb
# r : 反向的绝对位置emb
# r_w_bias :公式中的u
# r_r_bias : 公式中的v
# attn_mask : attention mask矩阵
# mems : memory
attentiton score
矩阵的生成
def rel_shift(x):x_size = tf.shape(x)x = tf.pad(x, [[0, 0], [1, 0], [0, 0], [0, 0]]) #第二维padding [qlen,klen,bsz,nhead]x = tf.reshape(x, [x_size[1] + 1, x_size[0], x_size[2], x_size[3]]) #reshape产生偏移x = tf.slice(x, [1, 0, 0, 0], [-1, -1, -1, -1]) #截取attention score矩阵x = tf.reshape(x, x_size)return x
Segment-level recurrence mechanism - Memory 代码解析
- 同样在解析代码前,先用图示展示Memory的实现过程
- 当前
为query,和
内积;
- 按照之前讲解的方式得到relative pos和
的内积结果;
- 得到attention score后,通过
得到attention score矩阵
;
- 当前
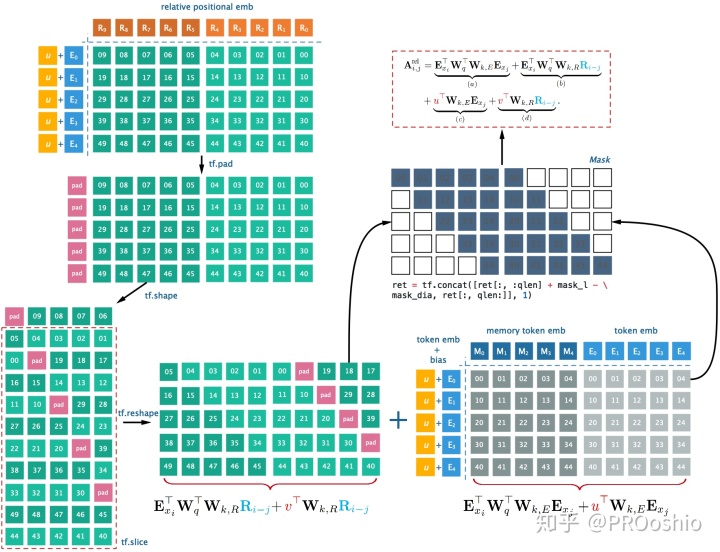
- 在tf中,_cache_mem(*)函数返回上一个
def _cache_mem(curr_out, prev_mem, mem_len=None):if mem_len is None or prev_mem is None:new_mem = curr_outelif mem_len == 0:return prev_memelse:new_mem = tf.concat([prev_mem, curr_out], 0)[- mem_len:]return tf.stop_gradient(new_mem)
- attentiton mask矩阵的生成
def _create_mask(qlen, mlen, same_length=False): #same_length : 每个token是否采用相同长度的attn length# 代码中train阶段为False 测试时是Trueattn_mask = tf.ones([qlen, qlen]) # 1: mask 0: non-maskmask_u = tf.matrix_band_part(attn_mask, 0, -1) #上三角 = 1mask_dia = tf.matrix_band_part(attn_mask, 0, 0) #对角 = 1attn_mask_pad = tf.zeros([qlen, mlen]) # memory的maskret = tf.concat([attn_mask_pad, mask_u - mask_dia], 1) #如果使token相同的attn_len,设置下三角maskif same_length:mask_l = tf.matrix_band_part(attn_mask, -1, 0)ret = tf.concat([ret[:, :qlen] + mask_l - mask_dia, ret[:, qlen:]], 1)return ret
relative pos encoding
完整代码
def rel_multihead_attn(w, r, r_w_bias, r_r_bias, attn_mask, mems, d_model,n_head, d_head, dropout, dropatt, is_training,kernel_initializer, scope='rel_attn'):
scale = 1 / (d_head ** 0.5)
with tf.variable_scope(scope):qlen = tf.shape(w)[0]rlen = tf.shape(r)[0]bsz = tf.shape(w)[1]cat = tf.concat([mems, w],0) if mems is not None and mems.shape.ndims > 1 else ww_heads = tf.layers.dense(cat, 3 * n_head * d_head, use_bias=False,kernel_initializer=kernel_initializer, name='qkv')# word线性映射r_head_k = tf.layers.dense(r, n_head * d_head, use_bias=False,kernel_initializer=kernel_initializer, name='r')# pos线性映射w_head_q, w_head_k, w_head_v = tf.split(w_heads, 3, -1)w_head_q = w_head_q[-qlen:] #将memory从query中剔除klen = tf.shape(w_head_k)[0]w_head_q = tf.reshape(w_head_q, [qlen, bsz, n_head, d_head])w_head_k = tf.reshape(w_head_k, [klen, bsz, n_head, d_head])w_head_v = tf.reshape(w_head_v, [klen, bsz, n_head, d_head])r_head_k = tf.reshape(r_head_k, [rlen, n_head, d_head])rw_head_q = w_head_q + r_w_biasrr_head_q = w_head_q + r_r_biasAC = tf.einsum('ibnd,jbnd->ijbn', rw_head_q, w_head_k)BD = tf.einsum('ibnd,jnd->ijbn', rr_head_q, r_head_k)BD = rel_shift(BD)attn_score = (AC + BD) * scaleattn_mask_t = attn_mask[:, :, None, None]attn_score = attn_score * (1 - attn_mask_t) - 1e30 * attn_mask_tattn_prob = tf.nn.softmax(attn_score, 1)attn_prob = tf.layers.dropout(attn_prob, dropatt, training=is_training)attn_vec = tf.einsum('ijbn,jbnd->ibnd', attn_prob, w_head_v)size_t = tf.shape(attn_vec)attn_vec = tf.reshape(attn_vec, [size_t[0], size_t[1], n_head * d_head])attn_out = tf.layers.dense(attn_vec, d_model, use_bias=False,kernel_initializer=kernel_initializer, name='o')attn_out = tf.layers.dropout(attn_out, dropout, training=is_training)output = tf.contrib.layers.layer_norm(attn_out + w, begin_norm_axis=-1)
return output